Proteins are biological nano-machines that power every living thing on our planet. While the variety of known proteins is extraordinary, all proteins consist of 20 simple building blocks called amino acids. The precise sequence of amino acids is encoded in our DNA and determines the biophysical properties of a protein. Depending on the sequence, amino acid chains fold in distinct 3D structures within a fraction of a second. It is this shape that allows proteins to carry out their highly diverse functions.
Protein prediction based on amino acid sequence
Knowing the folding properties of proteins is highly valuable for biomedical research. By comparing folding patterns, we are able to predict the function of a protein and test our assumption using biochemical assays. Small mutations in our DNA can have a detrimental impact on the efficiency of biochemical reactions catalyzed by proteins in our bodies and many diseases are either a direct result of misfolding, such as Alzheimer’s disease, or indirectly caused by changes in protein activity. The knowledge of the three-dimensional structure of a protein is therefore invaluable in designing novel drugs to modulate protein properties. Many recent advances in experimental procedures have improved our ability to determine the structure of biomolecules such as proteins (Figure 1), which are used to improve treatments for diseases such as cancer. However, finding the precise conformation of a protein is inherently difficult. Elaborate experimental methods such as X-ray crystallography and cryo-electron microscopy (cryo-EM) require highly purified protein samples and take up a lot of resources such as time and money to determine the structure of individual proteins. While we have made progress in reconstructing protein folding patterns in experimental settings, the unimaginable variety of proteins and the low-throughput of our gold-standard methods make it almost impossible to find the conformations for all disease-associated proteins.
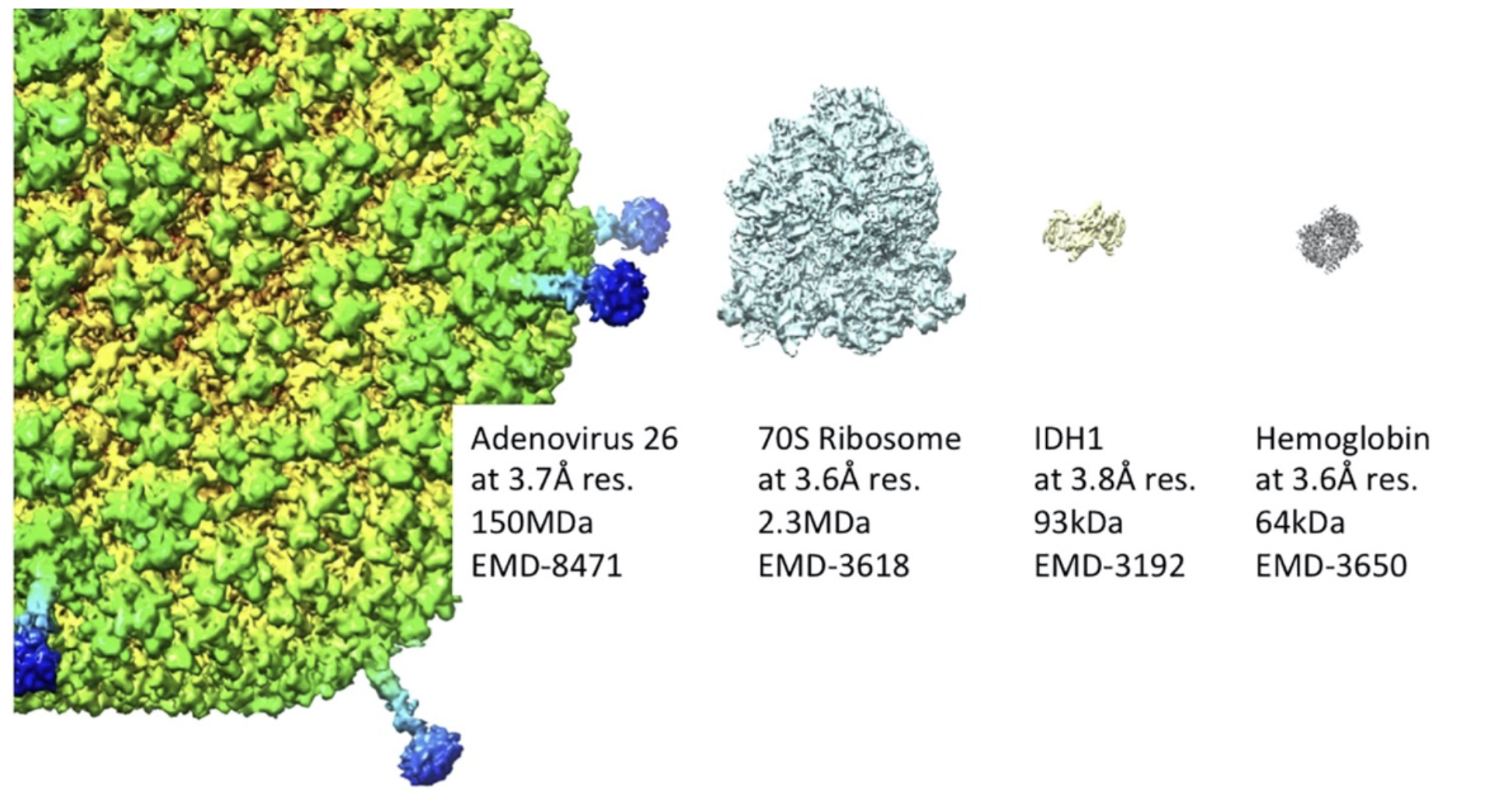
Figure 1:
Representative bio-molecules solved by cryo-EM at near-atomic resolution. Cryo-EM covers a wide molecular weight range of specimens, from protein complexes in the tens of kilo Daltons to large virus particles with hundreds of mega Daltons. Estimated resolution of the reconstruction, molecular weight and EM data base number are indicated for each particle (modified from Murata & Wolf, 2018).
Holy Grail in structural biology within reach
While the 3D structure of most proteins is unknown, the sequence of amino acids is relatively easy to determine by genetic analysis. It has been theorized already over 50 years ago that the structure of a protein should be guided by the amino acid sequence alone but many attempts in modelling protein folding have failed to deliver acceptable results that scientists can rely on in their downstream research endeavors. This is partially due to the incredibly high number of possible ways a protein could theoretically fold.
It is estimated that a typical protein has around 10300 (!) possible conformations.
The fact that most proteins fold into a precise conformation relatively quickly despite the astronomically high number of possible folds is known as Levinthal’s Paradox. The Holy Grail in structural biology has therefore been the development of a computational approach to determine the structure of a protein solely based on its 1D amino acid sequence.
Artificial intelligence (AI) can help predict the structure of proteins
The recent advances in artificial intelligence, however, have now solved this grand challenge in biology. Google-owned company DeepMind has recently revealed an algorithm, named AlphaFold, that is able to predict protein conformations based on sequence information alone. By combining principles of physics, biology and machine learning, DeepMind’s team devised a deep learning approach that accurately predicts protein structures. By training their system on over 170,000 experimentally determined protein structures (from open-source Protein Data Base, PDB) as well as a comprehensive database of known protein sequences (UniProt), AlphaFold is able to accurately predict 3D structures with an average error rate of less than 1.6Å, comparable to the width of an atom (Figure 2). This is a massive accomplishment in the field of computational biology and will have large impact on the life sciences and medicine.
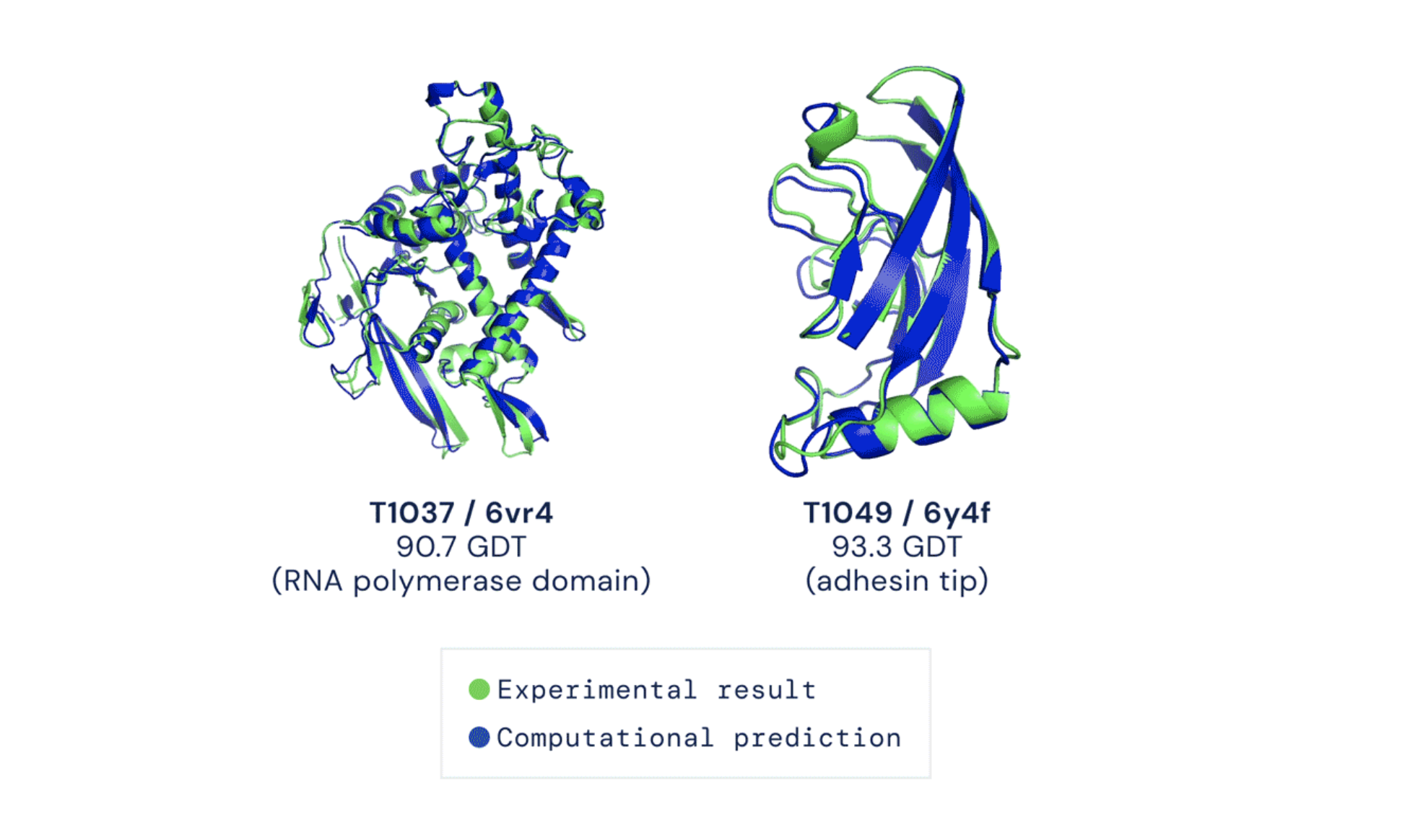
Figure 2:
AlphaFold predicts protein structures with high accuracy and an error of less than 1.6Å (0.16nm) on average (image credit: DeepMind).
Just the beginning of the AI revolution in science?
Algorithms such as AlphaFold have already started to change the way scientists work all over the world. The improvement of AI technology is highly promising in aiding research to become more effective, less reliant on model organisms such as mice, and faster than ever before. The potential applications for AI in research are just beginning to emerge. As a next step, AlphaFold could be further adapted to predict conformations of other biomolecules such as DNA and RNA, which will have great implications on the development of RNA-based therapeutics such as aptamers, oligonucleotides that can bind proteins similarly to small molecule drugs. Furthermore, by utilizing deep learning approaches it might be possible to design proteins from scratch able to bind specific drug targets or even design effective antibodies targeting emerging pathogens such as SARS-CoV-2.
The discovery of novel proteins not derived from any organism will not only revolutionize medicine but will also have an impact on patent law. AI-guided proteins designed to bind a desired drug target with the intention to treat diseases will create biomolecules that are completely artificial, similarly to small molecules commonly designed by the pharmaceutical industry. Human designed proteins produced from artificial DNA are patentable as they are not naturally found in nature.
References and resources:
Murata, K., and Wolf, M. (2018). Cryo-electron microscopy for structural analysis of dynamic biological macromolecules. Biochimica et Biophysica Acta (BBA) – General Subjects 1862, 324–334.
https://web.archive.org/web/20110523080407/http://www-miller.ch.cam.ac.uk/levinthal/levinthal.html
https://deepmind.com/blog/article/alphafold-a-solution-to-a-50-year-old-grand-challenge-in-biology